Why broken socio-economic forecasting needs an overhaul
Welcome 2022 with financial clarity! Discover 5 tips to organize your finances, optimize investments, and prep for market shifts.
%20(1).webp)
2020 was a year of unprecedented events, from the Australian wildfires which began the year to the COVID-19 pandemic which consumed most of it. Some of these events were predicted well in advance and represented intensifications of existing trends, particularly those concerning environmental disasters resulting from climate change. Others, however, were seen as “unpredictable” and defying of conventional wisdom pushed through official channels of expertise. To name but a few, the continued rise of the stock market, the increased pace of housing starts and sales despite the pandemic, and the so-called “K-shaped” economic recovery coming from it, went against the expectations of many institutional actors, suggesting a disconnect between institutional predictive analysis and what’s actually happening in the world.
In 2021, we are already seeing the effects of increasing unpredictability in the markets, with the rise of retail investors leading to a massive increase in the stock valuations of moribund companies such Gamestop and AMC Theatres. The disruptive influence of zero-commission stock trading apps and social media networks aside, these events have clearly caught institutional experts off-guard, resulting in massive losses at institutional hedge funds.
Even before the much more visible disruptions of the past year, conventional wisdom was being quietly upended through slower mechanisms. Take, for example, the fact that, pre-COVID, the Trump administration’s unconventional economic approach caused unemployment figures to reach record lows without causing the inflation predicted by most economists. Policymakers now, even those who opposed Trump on many other grounds, are currently in the process of sorting through just what implications this success may have for both general understanding of employment markets and how post-COVID stimulus might be formulated for maximum impact. The US shale oil boom, coming as it did after years of predictions on “peak oil” preceding it, can be seen as another example where conventional analysis did not take into account factors beyond a certain scope of investigation, which left out technological advances in oil extraction.

Source: https://www.sciencedirect.com/science/article/pii/S0306261918303428#f0010
One or two of these events could perhaps be dismissed as sui generis “black swans” which defy conventional explanations, but do not necessarily portend a broader shift in understanding. However, taken in combination, the events of past years and those which are likely to come in the new year testify to the need for new approaches to predicting events and trends in the world.
The causes of this need are important to understand because so much of the ability of policy makers, investors and companies to make effective decisions depends on what they believe will happen in the future and how accurate their foresight is. Decisions on investment, whether public or private, become increasingly difficult to predict returns upon in the absence of accurate data. Instead of relying on conventional, often lagging, metrics, professional investors are increasingly turning to unconventional and real time sources of data. This data can include internet traffics, search histories, foot fall, flight data, scientific and technical data streams related to natural resources and many more.
These data streams can be tremendously useful for a variety of purposes but come with a number of their own complications. For one, the sheer volume of available data requires curation in order to be useful or even comprehensible, due to problems of human comprehension past a certain scale. More importantly, the data on their own do not tell a compelling narrative and so require further analysis to be shaped into a course to guide action. In theory, this is what investment analysts and others who work in the world of predictive analysis are supposed to be doing with all indicators, whether conventional or not, but this introduces the possibility of interpretations being biased by a variety of factors. The individual experiences, expertise levels and social positions of analysts risks tainting the underlying data with their expectations. Indeed, the selection of particular types of data by itself introduces bias, as certain data are by nature excluded from consideration due to not appearing useful or relevant. As can be seen from the several examples above, data which are ultimately relevant to market trends were not considered relevant by actors within the market, which resulted in substantial financial losses for some (and substantial gains for those who were privy to this information). Whether the issue is the collection and validation of data, the scope of data considered in analysis or interpretation of what data ultimately reveals, bias affects the ability of analysts to produce accurate predictions based on the data they observe.
Three primary kinds of bias impact the ability of current prediction practices to “get it right”:
#1 Narrative Bias

Source: Federal Reserve Board
Humans are inherently pattern-seeking creatures and prone to seeing what “makes sense”, rather than what is actually true. Data without a narrative, or at least a set of patterns, is perceived to be simply random, meaningless information. Much of the work of analysis, therefore, is a sort of storytelling, where individuals tell themselves and others what data mean in order to give it force. We have a tendency to reject out of hand narratives that don’t “seem right” at face value, given our previous experiences or expectations. Just as much, we disregard outlier data when it doesn’t fit the narrative we want to construct. This does not necessarily make narratives false, but it does mean that they could be less-than-fully true, or true only in a qualified sense.
Further, as much as compelling storytelling can make truths have more of an impact, it can just as often give rise to falsehoods because they feed our common desire to make meaning out of chaos. Indeed, this impulse is what gives rise to conspiracy theories and other forms of counterfactual thinking. The large amount of misinformation and half-truths circulating on everything from election results to COVID-19 vaccines testifies to the fact that narrative has never been more powerful than now. The ability of individuals to connect in near-instantaneous fashion across the world amplifies both the positive and negative aspects of compelling narratives by increasing their ability to reach millions of people very quickly.
In terms of predictive analysis, this means that we should be wary of those who tell “just so” stories that make sense on the surface but lack sufficient data to back them up. Too many debates in economics, to choose one example, are based on theoretical models that make sense on paper or in a 101 classroom but do not bear out in the real world. When policymakers and other experts are beholden to these theories, they will continually shape the facts as they see them to fit their narrative expectations, rather than the other way around.
By contrast, we should also be open to narratives that do not “make sense” on the surface level or admitting that data often produces blurry results that do not point to particular conclusions, at least for the time being. Going further, we should challenge the notion that all models or forecasts need to be explainable in a conventional sense. The economy is widely complex and non-linear, and there are cases where following the data without explanation might actually produce greater predictive power. This is a key insight which can be gained from the trust that individuals place in many processes (such as the infrastructure of the Internet or autonomous vehicles), that they cannot fully explain on an individual basis. Nevertheless, we trust these systems because we have experience with them, trust the builders, and know that they are purpose functional.
The notion of getting away from narrative entirely is very challenging due to limits of human perception, but, widening the aperture of data we consider relevant to the narratives we construct can lead to better, more nuanced narratives that are closer to richness of the world of data. Building trustworthy, accurate systems can also increase the acceptability to individuals of predictions which may not have narrative explanations.
#2 Institutional Bias
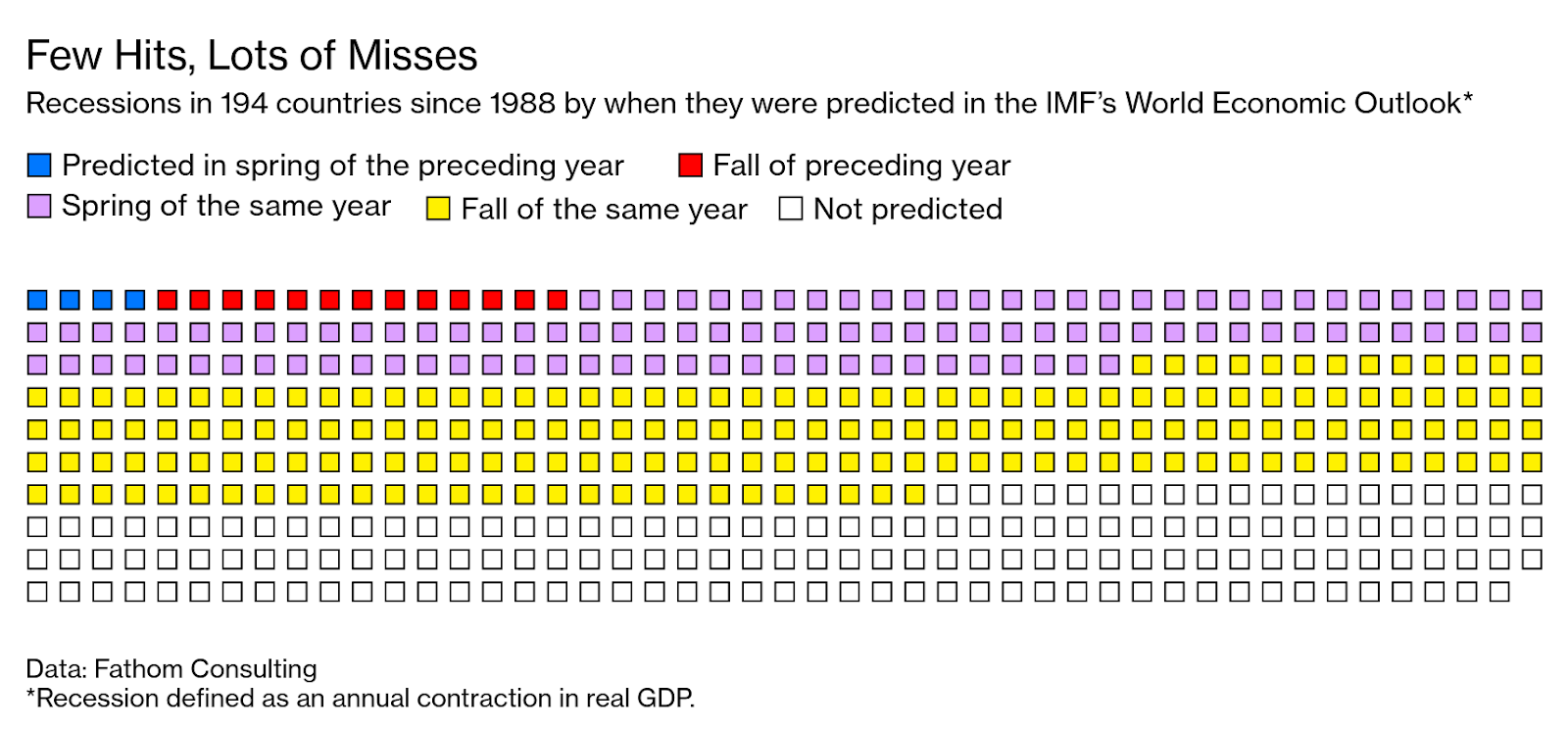
Many institutions that either provide data or interpret it are not necessarily in the business of providing unbiased analysis. There have been many examples of companies engaging data falsification in order to serve their own ulterior motives, from Enron to the 2015 Volkswagen emissions reporting scandal. That company-provided information should be taken with caution is now an article of faith amongst investors, and some laws have strengthened accountability and disclosure practices for publicly-held companies. But, even those institutions which are theoretically independent and “in the business” of providing assessments which are as accurate as possible often have perverse incentives. Excessively high credit ratings from major agencies contributed to the 2008 economic crisis, which in part may have been caused by the fact that those agencies are paid to consult by the companies and institutions they rate. Government institutions in countries such as China are understood to produce figures that should be taken with a grain of salt, but there are examples of misreporting closer to home from institutions expected to be more reliable as well. The recent scandal involving Florida’s COVID-19 case reporting is a vivid example of this.
Even respected international bodies such as the IMF and World Bank are not immune from institutional biases of their own. Critics of conventional international economic statistics note that they suffer from biases of expert attention and countability (meaning that more ephemeral economic fields such as knowledge production which make large contributions to future economic development are not counted). Further, the input statistics for such international organizations are generated by governments which have interests in presenting a more rosy picture of their economies (or, in some cases, a more dire one) than actually exists. This underlying data informs thousands of analysts, models, companies, banks, and institutions, which can permanently slant expectations moving forward. Even with some filters of expertise to root out truly outlandish claims, it is no wonder than a recent Bloomberg analysis found that the IMF forecasts underestimated GDP growth in 56 percent of cases and overestimated it in 44 percent since 1999.
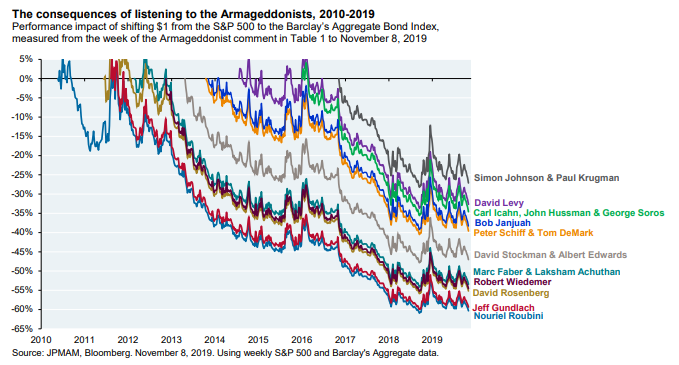
#3 Human Bias

Source:https://www.federalreserve.gov/newsevents/speech/yellen20150924a.htm
Individuals are powerfully informed by their own experiences and perspectives, even when they attempt to take themselves out of the question and have an “objective” look at the facts. When enough individual biases are collected together, they can often congeal into a sort of received wisdom or folk understanding of a problem set which suggests predictions or solutions which are far off the mark. Some of this bias tracks well-known social prejudices. For instance, research led by Sima Jannati at the University of Miami found significant “in-group” bias in financial market predictions on the basis of ethnicity, gender and political affiliation. Of course, the individuals and institutions performing the predictions would deny that anything of the sort is going on, and alternative factors may also play a role, but it is nevertheless disconcerting that underlying prejudices are getting in the way of accurate advice.
More subtly, the formative experiences of individuals often powerfully shape their perceptions of where the world is going and how to solve its problems going forward. Individuals in positions of political and economic power, those with the ability to truly “set the agenda”, are often older and less engaged with modern data trends and technological advancements. This can lead to a bias which could be called “past problem bias”, wherein individuals with the ability to direct solutions and predictions rely on their formative experiences (most often in youth) to guide action, rather than engaging with new problems. A good example of this is persistent fear of “stagflation” amongst many economists and policymakers who had their initial field experiences in the 1970s. Such fears may have appeared rational to those who professed them, but they have also likely impeded economic progress and led to reductions in unemployment due to worries about inflation -- which never in fact occurred.
Finally, humans are often fantastically bad at learning from their mistakes and errors. Good feedback loops for forecasting are quite rare and individuals seek to avoid cognitive dissonance between previous (inaccurate) forecasts and what actually occurred. Thus, they tend to misremember their estimates from previous situations and think of themselves as having more foresight than they actually do. These insights regarding the creation of feedback loops for forecasting were central to the book Superforecasting, whose lessons are only beginning to be applied at the institutional level.
Solutions from Global Predictions
It is likely impossible to ever craft a perfect model of economic markets or of other aspects of human behavior. Systems in the real world are infinitely complex and can vary wildly from moment to moment in ways that even the most sensitive tools are unlikely to be able to pick up. However, constructing better models with additional data inputs, presented in a way that is as compelling as existing narratives, can help to minimize bias within predictive models and provide better guidance for the future to those who depend on knowing what it holds.
By combining modern statistical forecasting, economic insights, and AI analysis with 22,278 data time series, models produced by Global Predictions’ proprietary software platform have shown a 32% improvement in accuracy against IMF World Economic Outlook forecasts in bias-adjusted backtests. In other words, even in a relatively early form, Global Predictions has bested one of the most respected and trusted names in international economic forecasting. This is because the model pursued by Global Predictions seeks to minimize these three key sources of bias. The platform’s use of AI and statistical forecasting acts to minimize intuitive human inputs, while widening the aperture of real time data sources makes missing a key input far less likely. Global Predictions’ single dashboard interface presents these insights in a clear and compelling fashion, while allowing for further analytical drill-down as needed. Most importantly, the only goal of the company is accuracy, not the various outside motivations which can mislead other forecasting which keeps the platform free of political or institutional influence.
2020 was a year like no other, and thus far 2021 looks set to continue that volatility. Greater predictive power is necessary for policymakers, companies, and investors to know how to navigate an unstable global reality. Recognition of key biases which hamper the creation of effective models is only the first step in moving away from an approach towards predictive analysis which relies on hunches, past actions, and gut instincts to one which is truly data-driven. The next step is building a model for the new era, which builds on the trend towards real time, unconventional data by applying rigorous analysis and intuitive presentation. This model will be judged solely on its accuracy, removing outside political and institutional influences in order to present users and analysts with the most accurate possible picture of the world as it exists and as it is going to be.
This is just what Global Predictions has done, and will continue to do.
How optimized is your portfolio?
PortfolioPilot is used by over 30,000 individuals in the US & Canada to analyze their portfolios of over $30 billion1. Discover your portfolio score now:
Analyze your entire net worth
360° portfolio analysis, AI Assistant, and personalized recommendations guided by our Economic Insights Engine.

How Tariffs Impact the Economy and Your Money
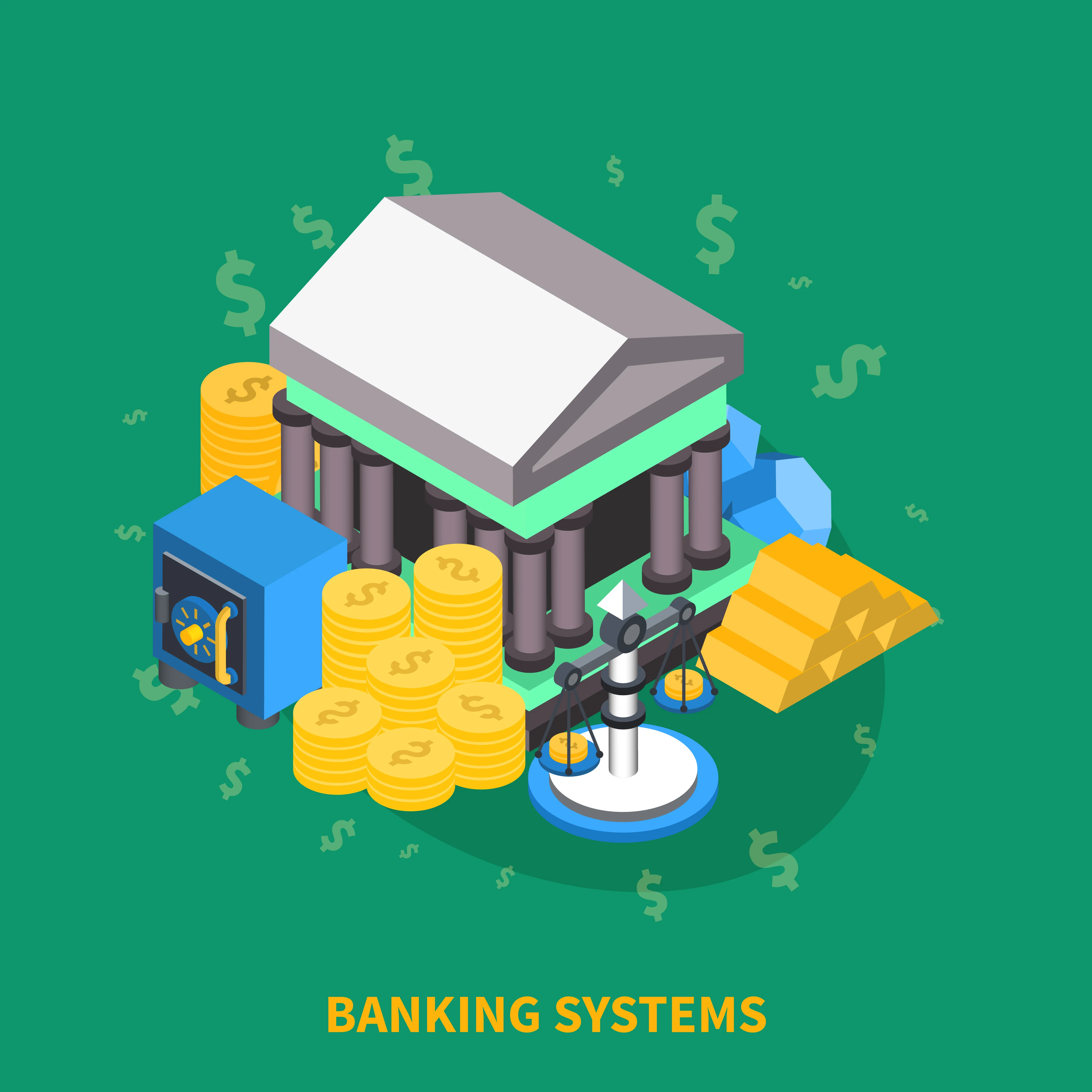
The Interest Rate Banks Charge Their Best Customers Explained
